Announcing the MICCAI Learn2Learn Challenge!
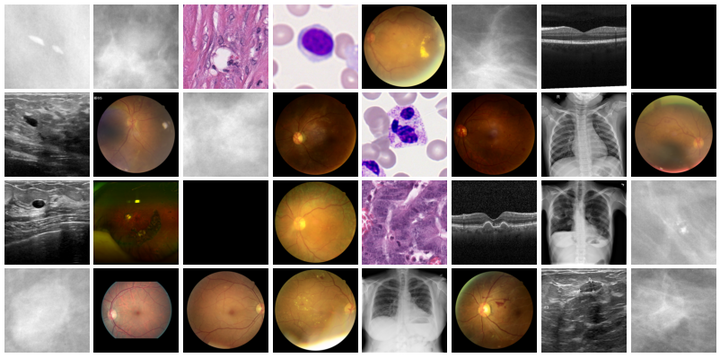
We are delighted to announce the MICCAI Learn2Learn challenge and the release of a novel dataset of datasets containing 28 medical classification tasks derived from 17 public datasets.
With the L2L challenge, we aim to bring cross-domain few-shot learning (CD-FSL) to the medical imaging domain. CD-FSL approaches such as meta-learning offer the potential to exploit similarities between different datasets to learn previously unseen tasks more efficiently. However, CD-FSL is underexplored in medical imaging.
To this end we release the novel MIMeta dataset comprising 28 unique tasks derived from 17 medical image datasets. This will allow you to create algorithms that learn new tasks with minimal annotated examples!
We believe that, the MIMeta dataset will be a valuable resource for benchmarking algorithms on a wide range of medical tasks with standardised data splits even beyond our challenge. Our overarching goal is to make CD-FSL accessible to MICCAI folks, and medical imaging accessible to ML folks!
Along with the dataset, we’re releasing two #PyTorch toolboxes for data handling and cross-domain few-shot learning to help participants kick-start their submissions, and make CD-FSL more accessible to the community!
Last but not least, the winners of the challenge will be awarded a 1000 CAD cash prize generously funded by ImFusion!
The L2L Challenge is co-organised by MLMIA lab members Stefano Woerner, Susu Sun and Christian Baumgartner, as well as by Thomas Küstner from the University Hospital Tübingen, and Hien Van Nguyen from the University of Houston.