Human-in-the-Loop Machine Learning Systems
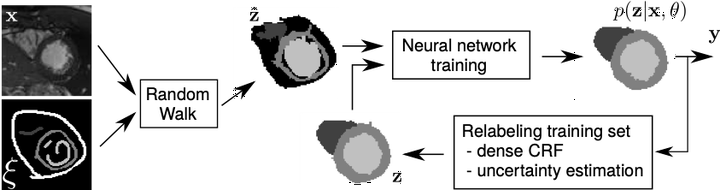
Humans are not only involved in obtaining the final prediction of a machine learning based system. Especially in medical contexts, human users are also involved in collecting and annotating the training data, and in continuously improving the model over time by adding particularly informative cases to the model. What is more, those steps can often only be performed by clinicians who often need to perform these tasks working after hours in addition to their clinical responsibilities. To address these topics we investigate optimizing annotation time by using weak and active learning techniques. In addition to placing humans in the training loop, for some applications humans must also be placed in the prediction loop. For instance the initial prediction of an algorithm, e.g. an organ delineation task or a radiotherapy plan, may not completely agree with the users expectations. The clinician may want to refine her query for the algorithm and obtain an improved prediction. How to integrate humans into the prediction loop is studied in the field of interactive learning.
Selected publications
- Yigit B Can, Krishna Chaitanya, Basil Mustafa, Lisa M Koch, Ender Konukoglu, Christian F Baumgartner, Learning to segment medical images with scribble-supervision alone, Proc. DLMIA 2018
- Krishna Chaitanya, Neerav Karani, Christian F Baumgartner, Anton Becker, Olivio Donati, Ender Konukoglu, Semi-supervised and task-driven data augmentation, Proc. IPMI 2019
- Lisa Margret Koch, Martin Rajchl, Wenjia Bai, Christian F Baumgartner, Tong Tong, Jonathan Passerat-Palmbach, Paul Aljabar, Daniel Rueckert, Multi-atlas segmentation using partially annotated data: methods and annotation strategies, Transactions in Pattern Recognition and Machine Intelligence (2017)